A/B testing. I can’t summarize what it is better than how Harvard Business Review did: “A/B testing, at its most basic, is a way to compare two versions of something to figure out which performs better.” The label “A/B testing” has been around for over a century, but let’s be realistic—the concept is much older than that. I’m sure people have been comparing things for way longer than a hundred years.
Fun fact: Where does the “100 years ago” come from? In the 1920s, statistician Ronald Fisher started testing crops with different types of fertilizer, forming a framework of principles and mathematics that is still used in today’s A/B testing applications.
But what does A/B testing have to do with e-commerce? Pretty much the same as it does with fertilizer–in this case, comparing two website variations with different characteristics (e.g, look and feel, functionality, etc.) to determine which yields a better conversion rate.
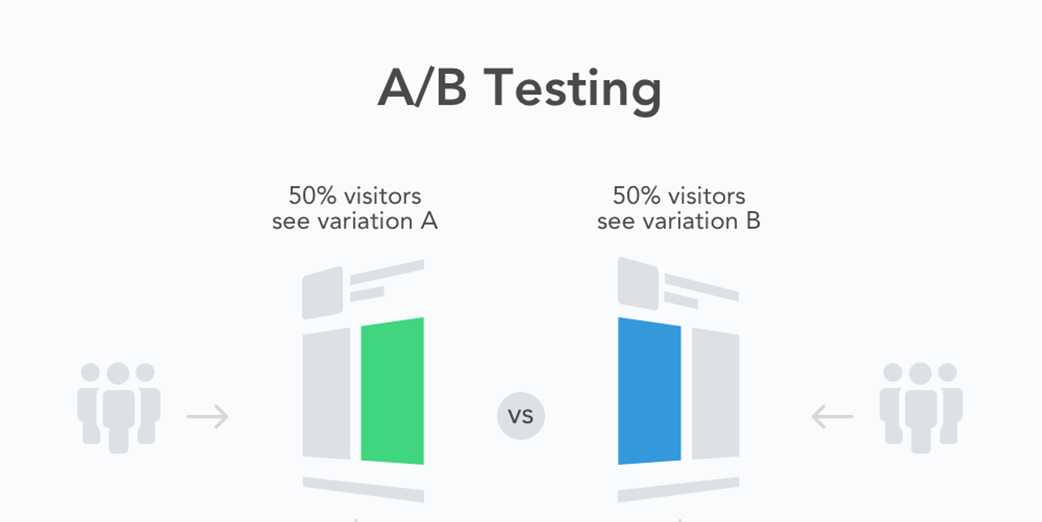
While conversion is typically the ultimate metric, there is other functionality that could be incorporated into an A/B testing structure, such as:
- Calls to action: For example, to call attention to an urgent message (e.g., “Only 1 room left!”), A/B testing can determine which of two colors and locations of a message might stand out more.
- User behavior: Are users more likely to convert if they view more unique product detail pages? A/B testing can offer two different user experiences to see which one is more effective.
- Sales Funnel: The further a user gets down the funnel, the more likely they are to convert—but how do we capture the few that make it to the cart but don’t buy? A/B testing can help identify those opportunities.
At a high level, conducting A/B testing is a simple series of steps. It starts with an idea, whether instinctual or data-informed, around which a hypothesis is created. In the case of A/B testing, the hypothesis involves answering more questions than “What’s the expected outcome?” In this step, determining who should be involved in the test and how long should the test run, etc. will inform the implementation. Which takes us to the next step, which is – probably not surprising – implementation.
There are different ways to implement A/B testing, so in this step there might be development work involved, event creation, and incorporating analytics. This is the step that often requires the greatest time investment, but once its complete, the fun part begins.
That brings us to the actual running of the experiment. This is the step that I get really excited about. Whenever I have A/B tests running, the first thing I do every morning (after I get my coffee) is look at my test results. Sometimes tests come in sooner, and sometimes tests will run for months before enough information is available to reach statistical significance: keep in mind that the minimum number of users needed to conduct effective A/B testing depends on the average for a specific website. The time it takes to hit statistical significance can be much longer for sites that experience less traffic, in general, than for those that are being used more heavily.
In the context of AB testing experiments, statistical significance is how likely it is that the difference between your experiment’s control version and test version isn’t due to error or random chance. For example, if you run a test with a 95% significance level, you can be 95% confident that the differences are real.
The final step is to form a conclusion. In short: Did A or B win? It can be easy to get emotionally involved in some of these tests—especially if the test is something that you had an instinctual drive to create. But at the end of the day, you have to trust the data, and in e-commerce testing, conversion is king.
There are so many questions to consider when determining whether a test was successful:
- Did it confirm your hypothesis?
- Was there an increase in expected metrics?
- Did it have any unforeseen negative impact on another area?
And there are so many metrics to consider when determining why a test won or lost: mobile versus web, OS or device type, geolocation, and so on. The hard truth is, according to Optimizely, a web-based analytics and A/B testing platform, A/B tests have only a 25% success rate.
A/B testing is everywhere
Whether you know it or not, you have participated in A/B tests, meaning you have shaped countless features, apps, behaviors, and experiences.
A/B testing can involve more than two options, as well. There are multivariate versions of A/B testing, often used most successfully in sites that experience a good amount of traffic. With multivariate testing, things can get pretty crowded, making it difficult to interpret the data, but tools like Optimizely help with segmenting the data and making it tangible.